Multi-Branch Attention Networks for Classifying Galaxy Clusters
Yu Zhang, Gongbo Liang, Yuanyuan Su, Nathan Jacobs. ICPR 2020
University of Kentucky
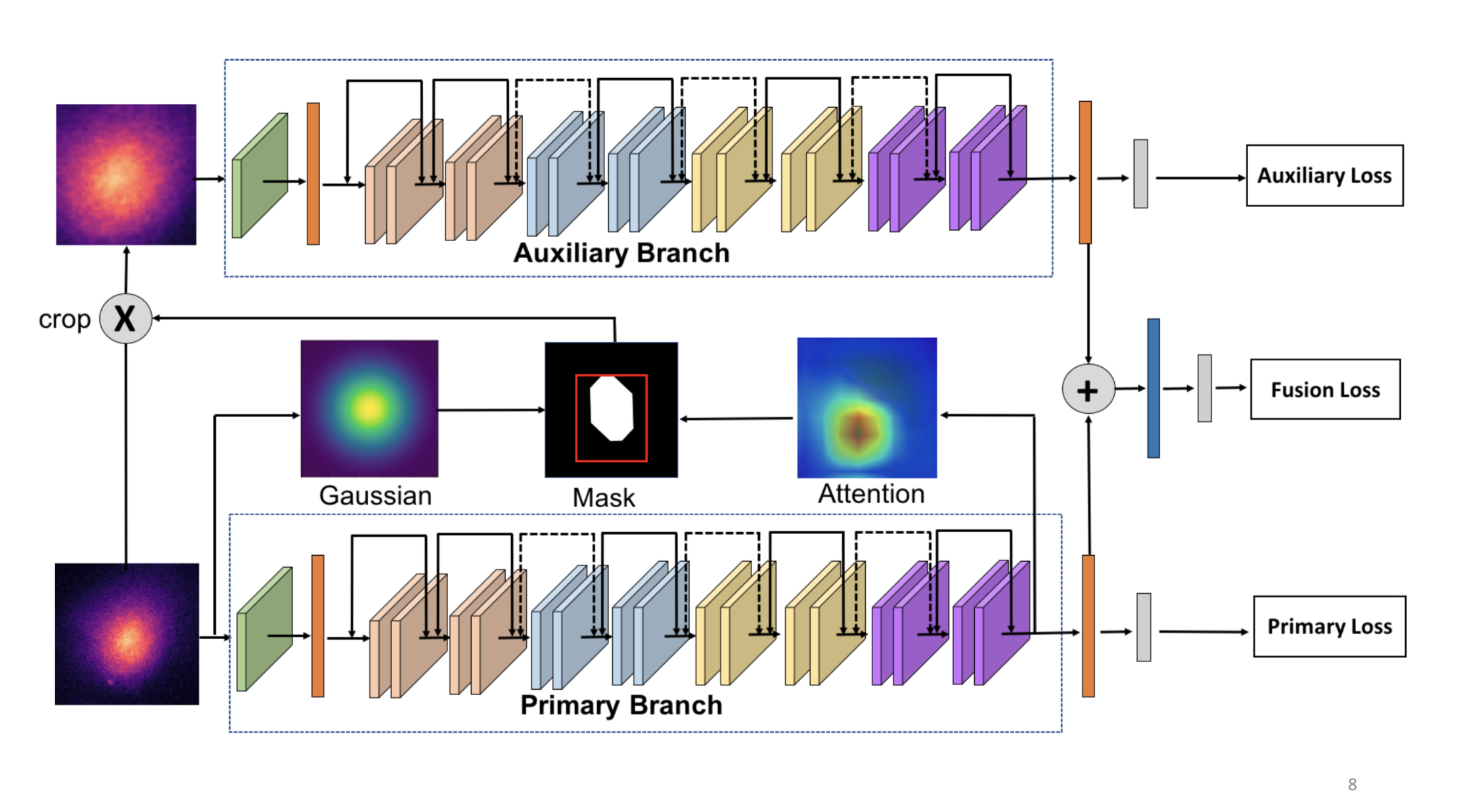
This paper addresses the task of classifying galaxy clusters, which are the largest known objects in the Universe. Galaxy clusters can be categorized as cool-core (CC), weak- cool-core (WCC), and non-cool-core (NCC), depending on their central cooling times. Traditional classification approaches used in astrophysics are inaccurate and rely on measuring surface brightness concentrations or central gas densities. In this work, we propose a multi-branch attention network that uses spatial attention to classify a given cluster. To evaluate our network, we use a database of simulated X-ray emissivity images, which contains 954 projections of 318 clusters. Experimental results show that our network outperforms several strong baseline methods and achieves a macro-averaged F1 score of 0.83. We highlight the value of our proposed spatial attention module through an ablation study.